Amid the growing number of patients with cancer, ongoing nursing shortages, high staff turnover rates, and nurses’ general desire for more flexible and balanced work, innovative oncology nurse managers are turning to technology and data as creative—even critical—tools for effective staff scheduling.
Acuity-Based Staffing Models
ONS member Patrick Evans, RN, BSN, MBA, senior nurse manager in the hematology and hematological malignancies department at Fred Hutchinson Cancer Center in Seattle, WA, managed the ambulatory hematologic malignancies department during the COVID-19 pandemic. Like many of his peers around the world at the time, he struggled with challenges with a growing department to meet the need for ambulatory options for immunocompromised patients, nurses becoming burned out, and ultimately recruiting to fill both gaps. In an effort to understand why many staff were leaving the profession or looking for different roles, Evans led an initiative to identify better ways to equip the team, serve patients, and support nurses.
Evans said that the patient population at Fred Hutch is complex and requires varying levels of expertise and time based on individual patients. The provider group covers six different hematologic diseases and malignancies with varying acuity levels. Nurses were expected to care for patients in all disease states, although physicians are more specialized. Evans and his team proposed positioning nurses into groups similar to their physician model “so they could also become experts and really well-versed and well-rounded in their education for those disease groups,” Evans said. “Categorizing nurses into specialties within the hematology group was really important.”

Evans partnered with the Fred Hutch nurse education department to conduct literature searches and research acuity models, but most of the examples they found were from infusion centers rather than holistic clinical settings like theirs.
“Our nurses are not actively engaged in putting IVs in, doing hydration, and administering chemotherapy. They’re focused on the global scope of care—partnerships with physicians, advanced practice providers, and schedulers. They are doing more of a holistic approach, answering supportive care needs, and coordinating all of our efforts for the patient. They are incredibly busy, but they’re not doing that physical, hands-on care,” he said. “There weren’t acuity models based on that.”
With no blueprint to follow, the team conducted interviews and analyzed patient volume. Ultimately, Evans and team decided that, instead of looking at the number of physicians per nurse or the number of patients per physician, they would determine the appropriate nurse-to-patient ratio. “We were able to create nurse assignments by total volume of patients on individual panels,” he said.
Incorporating both qualitative and quantitative measures of acuity from their electronic health record’s (EHR’s) in-basket usage, telephone calls, scheduled nurse visits, provider clinical support, coordination of treatment for urgent add-ons, admissions, emergency department use, and total nurse-patient panel size, they used 100 “active” (e.g., acute care) patients and another 100 “inactive” (e.g., surveillance) patients per nurse to identify patient panel limitations. Evans’s team continuously monitors the data and flags any numbers that vary by 25% to reassess and evaluate the need for additional changes.
After establishing those requirements, Evans and his team noticed that “we were short-staffed to be able to meet our own standard we had just created.” They pivoted and developed a business plan to request several nursing positions to meet the level set.
Their request was granted, but Evans said it would not have been possible without a supportive executive leadership group. His advice to others in a similar situation? “One of the key pieces is that you can do all the work, but engaging with your stakeholders and having a group of stakeholders that care that much is extremely beneficial.”
Fred Hutch’s new system allowed Evans’s team to move to 10-hour shifts, four-day workweeks, and a one-day-a-week work-from-home option. In a two-year follow-up analysis, Evans reported that his institution reduced its number of travel nurses from eight or nine to none. He also reported that their current turnover is very low, with the exception of nurses growing their roles within the department, and a “new to specialty” nursing role that has provided attractive recruitment opportunities for nurses to learn more and advance their skills within oncology.
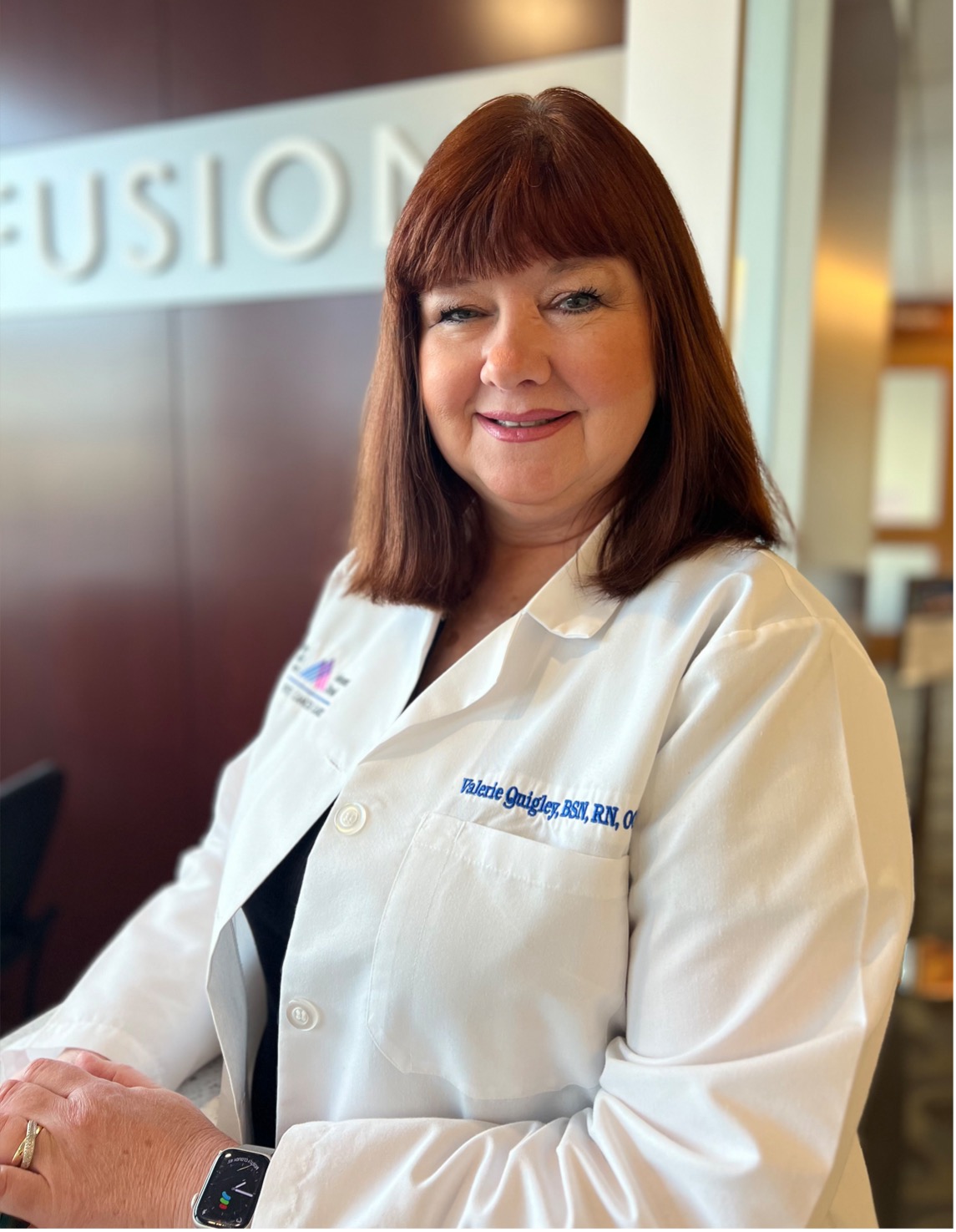
iQueue and Other AI Nurse Scheduling Tools
Artificial intelligence (AI) is influencing all areas of society, including nurse scheduling, with several tools on the market. Valerie Quigley, RN, BSN, OCN®, HN-BC, infusion manager at the Valley Hospital in Ridgewood, NJ, recently helped her organization to implement LeanTaaS’s iQueue and Nurse Allocation tools.
Like Evans’s model, iQueue centers on acuity levels, which are critical in Quigley’s busy community-based cancer center that has a limited number of chairs but a variety of treatments. Per day, the Valley team treats about 75–80 patients with an average of 12 nurses. About half of the patients are receiving chemotherapy, with the remainder receiving blood transfusions, hydration, other medication infusions, and injections. Although their number of patients continues to grow, the center is not able to increase beyond its 24 chairs. Using those chairs efficiently is critical.
Quigley said that iQueue and Nurse Allocation has optimized both space (i.e., patient scheduling) and staffing (i.e., care hours).
“Nurses are used to counting patients and not looking at patient care hours,” Quigley said. “The nursing allocation takes nursing care hours into consideration.”
Valley Hospital uses the Meditech EHR, which integrates with iQueue to assess patient volume and appointment types over the previous year and then builds appointment types for future scheduling. Patients are scheduled in specific slots of 30–60 minutes, 61–90 minutes, 91–120 minutes, and 240+ minutes.
iQueue’s embedded Nurse Allocation tool uses the patient schedule to identify nurses’ schedules. First, the manager enters the nurses for the day with their start and end times (based on whether they work 8-, 10- or 12-hour schedules). Then, the manager can simply “Run Allocation” to let the system balance the schedule.
Valley Hospital worked with its nurses and the vendor to customize the system to meet their needs. For example, they were able to color-code new patients who may require extra time. They also enabled staffing managers to view patients’ names in one view and appointment times in another, which allows the charge nurse to move patients in the allocation if needed. As the manager, Quigley can look ahead to identify staffing needs or determine if they can accommodate another patient. The charge nurse can look at the allocation to see the best time and staff available.
Implementing the tool did require some trial and error. “You need to give it a little time,” Quigley said. “We needed to frequently review our data and adjust our chair times accordingly.”
Getting nurses to buy into the process was the biggest challenge, Quigley said, mostly because everyone was learning the new system and how it worked. For example, “assignments were definitely challenging in the beginning until we knew what we knew,” Quigley said. “We learned that the less we manipulated our assignments, the better they were balanced.”
Vendor staff worked with the center’s nurses to address concerns and adjust the tools based on feedback. They also conducted pre- and postimplementation surveys, which found overall satisfaction and some areas for additional improvement.
“AI does have limitations,” Quigley said. “For example, you can’t take into account if a patient had a previous reaction or needs additional time. This is when you would have to manually move patients.”
Quigley said that patients also needed time to adjust to the new system. For example, they may have been used to coming at a specific time that was no longer available. To address this, Quigley developed a script for schedulers to explain why the changes were being made and how they would enable them to provide the best care to patients.
Quigley acknowledged that LeanTaas is costly; however, she said that the efficiencies gained both in terms of patient and chair turnover and scheduling compliance helped justify the costs. Quigley’s team identified and shared metrics that demonstrated those efficiencies, which helped them uncover things like bottlenecks, overall wait times, and wait times for pharmacy services. Managers could see which providers run behind on appointments to determine how long to allow between provider and infusion appointment times.
For others interested in incorporating AI into their scheduling, “staffing up at the beginning would be helpful, if at all possible,” Quigley advised. “Nurses also need to be involved in the planning and continued use of the product. They are the front line and have great input.”
She added that without proper programming, AI can’t take into account if a patient requests a specific nurse, has reacted frequently, or requires a new treatment. “You have to work with it, not against it,” she said.